4 min read
AI in Finance: Transforming the Industry with Opportunity and Caution
The advent of artificial intelligence (AI) has reshaped countless industries, but few have felt its impact as profoundly as finance. From transforming investment strategies to automating routine tasks, AI has introduced new efficiencies, greater accuracy, and valuable insights. Yet, while AI in finance promises a wealth of opportunities, it also brings with it unique risks and challenges. AI has changed the financial landscape, but let’s discuss the dangers of over-relying on it, and what makes for robust, reliable AI that can genuinely enhance financial decision-making.
How AI Has Changed Finance
Automated Trading and Investment Strategies
AI's impact on trading is one of the most significant changes in finance. With the ability to analyze vast amounts of data at remarkable speeds, AI-driven trading algorithms can make decisions faster than any human ever could. Algorithms such as those employed in high-frequency trading (HFT) detect patterns, make decisions, and execute trades in milliseconds, maximizing profit opportunities that would be unavailable in a manual trading setup.
Robo-advisors, which use AI to provide automated, algorithm-based portfolio management advice, are another area where AI has transformed investment. Many individuals who previously couldn’t access personalized financial advice now have tailored investment portfolios based on their goals, risk tolerance, and timelines.
Enhanced Fraud Detection and Risk Management
AI’s ability to recognize patterns and anomalies is invaluable in fraud detection and risk assessment. By analyzing behaviors and spotting irregular transactions, AI-powered systems can quickly identify and prevent fraudulent activity. This doesn’t only protect individual accounts but also adds a layer of security for entire financial institutions. Machine learning models can analyze historical data to predict and quantify risks, helping companies prepare for market changes and minimize potential losses.
Customer Service and Personalization
AI-driven chatbots and virtual assistants have transformed customer service in the financial sector. From handling routine customer inquiries to providing personalized advice, these tools allow banks and financial institutions to offer around-the-clock support while freeing up human agents for more complex tasks. Beyond customer service, AI has enabled personalized financial products by analyzing user data and providing tailored recommendations, making finance more accessible and customer-centric than ever before.
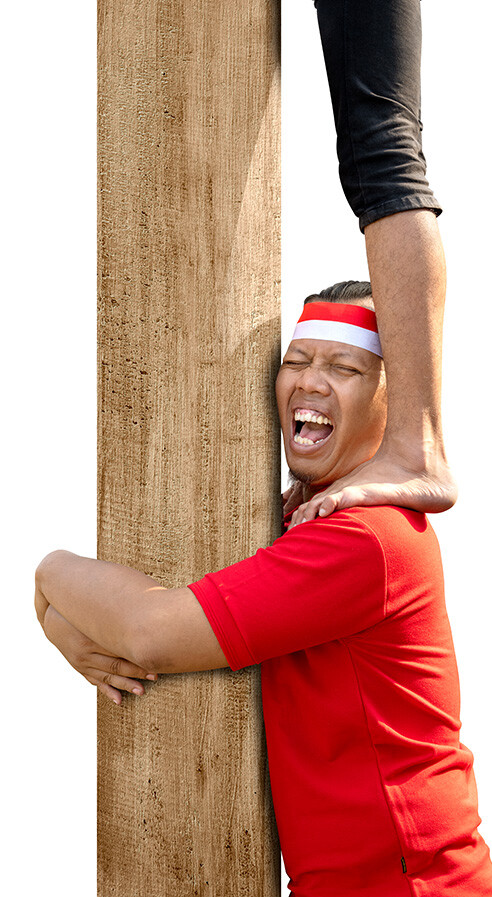
Dangers of Leaning Too Heavily on AI in Finance
Over-reliance on Algorithms Can Lead to Market Instability
While algorithms allow for fast, efficient trading, they also pose risks when markets shift suddenly. Algorithm-driven trading operates based on preset rules and historical data, which means these systems may not respond well to unprecedented events. If multiple trading algorithms react to the same anomaly, they can trigger a rapid sell-off, leading to market instability or even crashes. A notable instance of this was the 2010 "Flash Crash," where algorithmic trading contributed to a sudden and significant market drop in just minutes.
Data Quality and Bias Issues
AI models are only as good as the data they’re trained on. Poor-quality data can lead to flawed insights, and biased data can reinforce and even amplify those biases. For instance, if an AI system is trained on historical data that includes biased lending practices, it may replicate those biases, unfairly denying loans to certain groups. This is a serious concern, as biases can undermine fairness in lending, hiring, and even fraud detection.
Lack of Transparency and Explainability
A major challenge in relying on AI is the “black box” nature of many algorithms. These models can make complex calculations and predictions that aren’t easily understood, even by the experts who designed them. In finance, this lack of transparency is especially problematic. If an AI model recommends a high-risk investment strategy without clearly explaining the underlying reasons, clients may follow the advice without fully understanding the potential pitfalls. Regulators and financial professionals are pushing for AI models that are more transparent and explainable, but the industry still has a long way to go.
Cybersecurity and Privacy Risks
AI-driven financial services rely on vast amounts of sensitive data. As AI collects and analyzes this information, it creates new security vulnerabilities. Cyberattacks targeting financial data are on the rise, and breaches can lead to financial losses, identity theft, and reputational damage. Additionally, AI systems need access to customer data to deliver personalized services, which raises privacy concerns. Striking the balance between personalized services and data security remains a critical challenge for financial institutions.
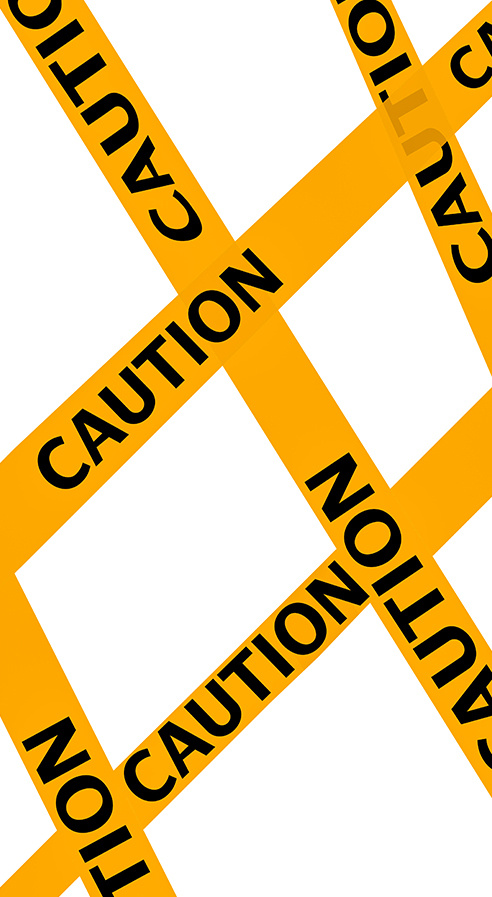
Characteristics of Good AI in Finance
Accuracy and Reliability in Data Collection
High-quality AI systems must start with high-quality data. Good AI in finance relies on clean, comprehensive, and current datasets. Financial data can come from various sources — transaction histories (something our own Botkeeper Infinite platform uses to inform its transaction categorization models!), social media sentiment, economic indicators, and more — and the ability to pull in reliable data is key. Effective AI systems have mechanisms for cleaning, sorting, and verifying data to ensure accuracy. Reliable data input means more reliable output, giving financial professionals confidence in the AI-driven insights they receive.
Transparency and Explainability
A good AI model in finance should be transparent and explainable. Financial professionals need to understand the reasoning behind the AI’s recommendations, whether it’s an investment opportunity or a risk assessment. Explainability is also critical for regulators, who may need to review AI models to ensure they comply with industry standards. Transparent AI doesn’t just foster trust among users — it’s essential for ethical, compliant decision-making.
Adaptability to Changing Market Conditions
The financial markets are dynamic, and a good AI model must be able to adapt to new information and market changes. A robust AI system should have mechanisms for continuous learning, allowing it to update its parameters as new data becomes available. For instance, if economic indicators suggest an approaching recession, an adaptable AI should adjust its models to account for this shift, ensuring that its predictions remain accurate in volatile markets.
Ethical Considerations and Bias Mitigation
Ethical AI in finance is a growing priority, especially as more decisions, such as loan approvals and investment advice, are driven by machine learning models. A good AI model actively identifies and mitigates biases, creating fair and inclusive financial services. This can be achieved by training models on diverse data and regularly auditing algorithms to detect and address potential biases. Ethical AI prioritizes fairness, ensuring that all customers are treated equitably, regardless of background.
Data Privacy and Robust Security
Given the sensitivity of financial data, good AI in finance must prioritize privacy and security. Financial institutions must adopt AI models that encrypt data, monitor for suspicious activity, and comply with data protection regulations such as GDPR or the CCPA. With cybersecurity threats on the rise, AI must include advanced security features to safeguard against breaches and protect user privacy. Customers need assurance that their data is in safe hands, and a secure AI system is the foundation of that trust.
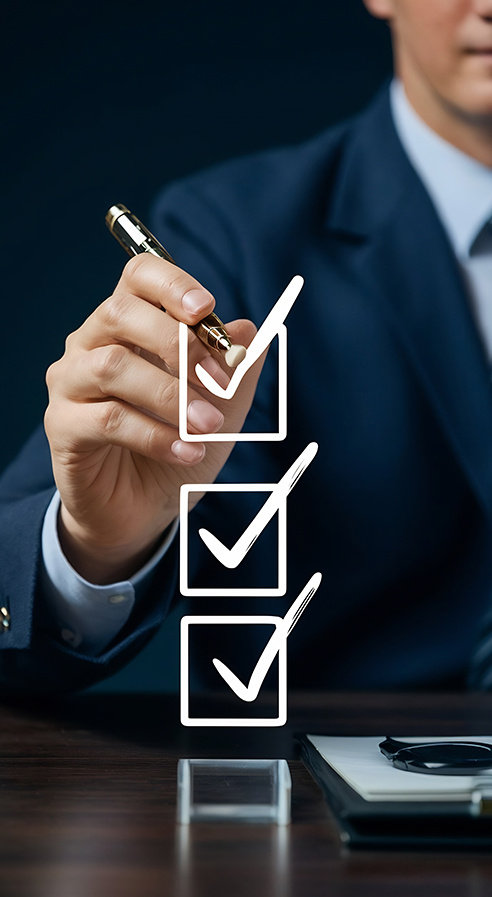
Moving Forward with Caution and Innovation
AI in finance has opened up incredible opportunities, making it possible to process vast amounts of data, enhance customer service, detect fraud, and personalize financial products. But the power of AI also calls for responsibility. Over-reliance on AI without understanding its limitations can lead to market disruptions, ethical pitfalls, and security concerns. Good AI in finance is not just about leveraging data; it’s about creating transparent, adaptable, ethical, and secure systems that serve everyone fairly. As financial institutions continue to embrace AI, balancing innovation with caution will be key to building a sustainable future in finance.
At Botkeeper, we promote responsible, effective AI that allows a human to enter the loop to intervene at any step. This ensures that your clients’ bookkeeping has what you deem to be the right amount of human oversight and involvement in the process. Check it out today to see how Botkeeper Infinite can increase your accuracy and efficiency, while helping make sure you have clean data and accurate financial reports.